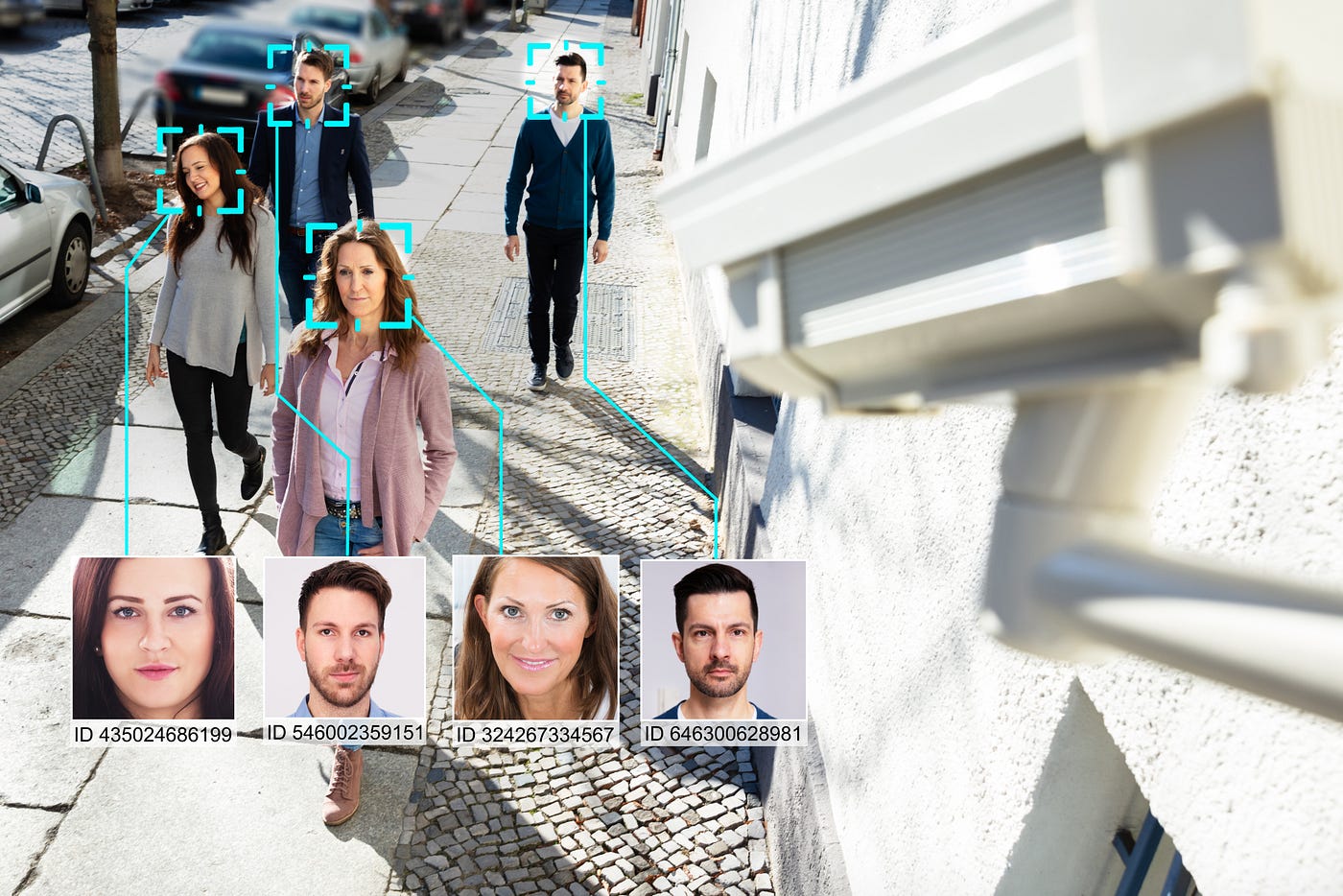
The emergence and evolution of information science have been one of many largest impacts of know-how on enterprises. As the online world retains rising and getting aggressive, there’s a dire want for companies to study as a lot as they’ll about their shoppers and the patterns impacting gross sales and income. Briefly, there’s a have to implement applied sciences that may simplify knowledge gathering, assorting, and managing, now greater than ever.
That’s the place Machine Studying (ML) is available in, the bleeding-edge know-how that’s garnering a lot consideration. However regardless of being a coming-of-age Twenty first-century know-how, ML stays a largely misunderstood space. Non-technical folks typically confuse it with Synthetic Intelligence (AI).
And that consists of our first level of demystifying Machine Studying. Sure, that’s what we’re going to do on this article. We’re going to demystify ML, particularly for the budding enterprise’ Beethoven(s) who’re inclined in the direction of inculcating this know-how into their enterprise apps and operational techniques however lack a transparent concept.
ML is an unlimited theme and given it has a well-entailed technological facet and naturally, a large spectrum of commercial advantages, we are going to cowl the matters which are required to have a very good grasp of it together with its many benefits. So let’s start with the obvious query.
Machine Studying is part of AI that includes using computational statistics, algorithms, and sensible mathematical optimization to make software program techniques smarter and extra correct at predictions. By gathering historic knowledge (each labeled and unlabeled knowledge) and analyzing it with the assistance of algorithms, the software program could make categorized predictions offering helpful insights for companies. Examples of utilization of ML embrace speech recognition, automated question responses, e-mail filters, and refined search engine outcomes.
The time period Machine Studying was coined by Arthur Samuel in 1959 whereas he was working at IBM. In line with him, the chief function of ML was to simplify and automate operations that granted computer systems their potential to study with out express programming and enhance the general person expertise.
As new apps, companies and applied sciences hold rising, there may be, certainly, a dire want to reinforce person expertise, and ML is doing fairly properly in that division. In truth, the survey stories revealed on ML by the German firm Statista forecast that by 2025, the ML market is estimated to develop from 22.6 billion U.S. {dollars} to nearly 126 billion U.S. {dollars}. It additionally states that ML constitutes the most important section of the AI market.
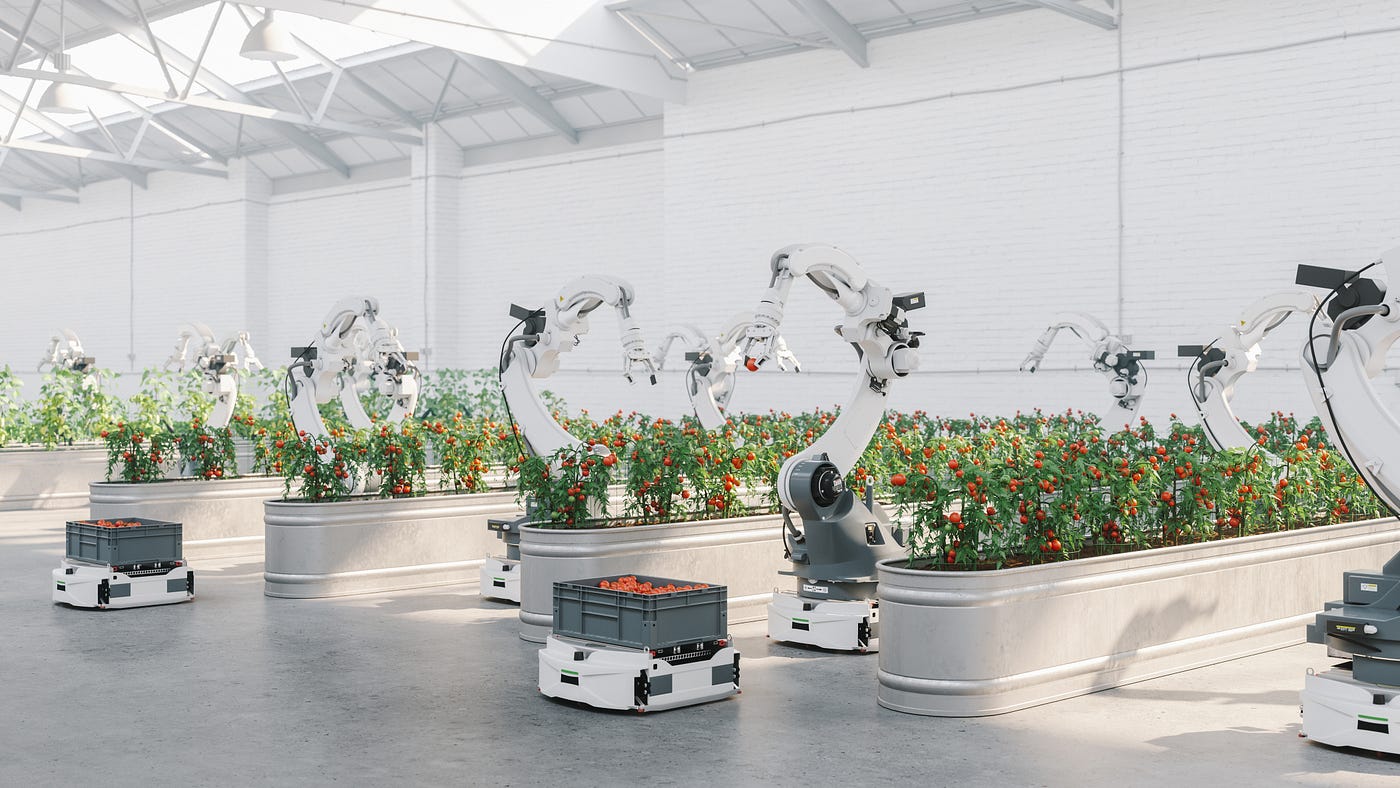
Identical to an app, a web site or an eCommerce portal, ML additionally capabilities on the buildup of various instruments and applied sciences. Essentially the most primary device that drives ML is an algorithm. When customers give their inputs, the ML algorithms monitor the info and attempt to hint the patterns, and reply accordingly (question responses and search key phrase recommendations).
ML mainly includes two sorts of knowledge — labeled and unlabeled. A labeled set of information is one by which knowledge samples are tagged with informative labels. For instance, for search options that allow looking out with a photograph, labeled knowledge might include an array of tagged photographs like home items, attire, identify of fruits, vegetation, and many others. So when a person scans an image of a blender, the algorithm instantly identifies the enter based mostly on the labeled knowledge and returns filtered outcomes.
Unlabeled knowledge alternatively is the one the place knowledge samples aren’t tagged. On this case, the ML mannequin has to go consider each bit of the given enter. For instance, if a person enters the image of an apple, but when it’s unlabeled, then the algorithm will consider each facet of the image like its coloration, form, and different traits to find out the fruit and return correct search outcomes.
Machine Studying fashions are categorized into 4 broad classes relying on the kind of dataset and the corresponding algorithm designed for job automation and knowledge classification. Let’s have a fast look.
1. Supervised Studying
Supervised studying is the most typical ML mannequin and contains engaged on labeled knowledge. The algorithm designed for supervised studying is educated to map the inputs and produce related outputs. Because the knowledge right here is already educated or supervised to study, predict and return anticipated outcomes, therefore the time period supervised studying. An instance of supervised studying is sorting emails into correct classes based mostly on their senders. That’s why your Gmail app is ready to obtain and kind emails into Main, Social and Promotions.
2. Unsupervised Studying
In an unsupervised studying mannequin, the algorithm has to coach itself by analyzing, processing and clustering unlabeled knowledge. Which means the algorithm has to iterate the inputs and uncover the hidden patterns within the datasets with out express programming. Each time the algorithm comes throughout a brand new set of information, it tries to establish the commonalities of the given knowledge and return outcomes accordingly.
A web based retail app units a very good instance of unsupervised studying the place the app classifies recommendations based mostly on the profile of customers, their searching patterns and bought objects.
3. Semi-supervised Studying
This ML mannequin blends the methods of the above two. A semi-supervised studying mannequin is educated through the use of a smaller set of labeled knowledge however giving it sufficient freedom to discover the brand new items of information and develop an understanding of its personal.
In truth, the labeled dataset of the algorithm offers route and empowers it to extract data from the bigger set of unlabeled knowledge. The sensible purposes of this mannequin are present in speech evaluation apps and in internet content material categorization.
4. Reinforcement Studying
Reinforcement studying is one other fashionable ML mannequin and works on an idea just like supervised studying. However as an alternative of utilizing labeled datasets, the mannequin is made to study by way of trial and error. The algorithms designed for this mannequin make use of dynamic programming methods. Some gaming apps use this studying mannequin whereas enjoying in opposition to a human opponent. Autonomous autos additionally make use of this studying mannequin.

It’s no secret that ML is a ubiquitous know-how. From the second you open your favourite procuring app to the time while you’re utilizing a textual content editor, it’s proper there. ML delivers a singular expertise to customers and on the identical time empowers companies with actionable knowledge and insights.
It holds immense potential for companies, particularly start-ups that may affect their potential clients with personalised advertising and marketing, enhance gross sales by realizing the place to focus, and deal with the under-performed areas. A number of the enterprise advantages of ML embrace:
- Making knowledgeable and higher selections with the assistance of correct knowledge
- Personalised product suggestions and elevated buyer satisfaction
- Help in making dynamic pricings like value hikes in automobile rental apps throughout poor climate situations or deciding on product pricing after comparability with native and worldwide manufacturers
- Streamline manufacturing by having higher forecasts of market demand and provide
- Elevated effectivity and productiveness with automated operations and discount in manufacturing defects
- Environment friendly monetary administration with correct estimations of enterprise bills and price evaluation
Machine Studying has come a far manner however it’s nonetheless evolving, and it’s far more difficult in technicalities. Positive it may assist start-ups make sensible selections and assist with useful resource administration with correct predictions, however to make sure its optimum utilization, one might want to rent devoted builders who’re well-versed with the know-how and know what is going to work for what. Solely then can one reap the various advantages of this modern-era tech.